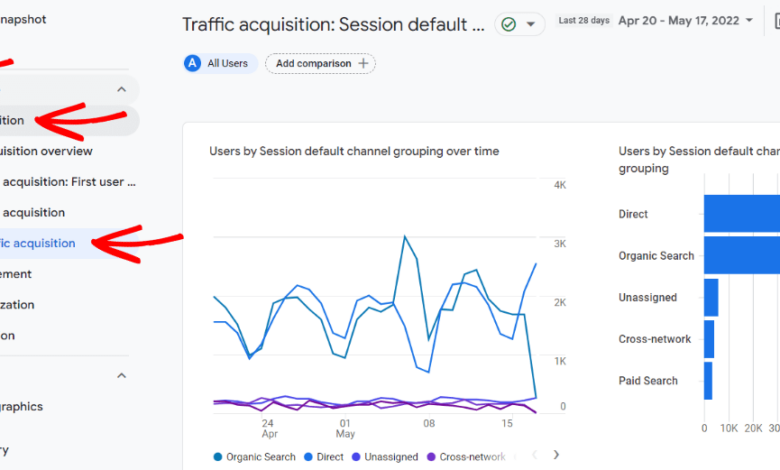
Is Your Analytics Referral Traffic Lying to You?
Is your analytics referral traffic lying to you? That’s a question more website owners should be asking! We all rely on analytics to understand where our visitors are coming from, but sometimes those numbers tell a misleading story. This post dives deep into the murky world of referral traffic, exploring how to identify deceptive sources, spot manipulation, and ultimately, get a clearer picture of your website’s true performance.
We’ll uncover sneaky tactics used to inflate referral numbers and reveal strategies to improve the accuracy of your data. Get ready to become a referral traffic detective!
We’ll cover everything from identifying trustworthy referral sources and analyzing traffic quality to investigating discrepancies in your data and implementing better tracking methods. We’ll even look at how to visualize your referral data to spot trends and anomalies – no more squinting at spreadsheets! This isn’t just about numbers; it’s about understanding your audience and making data-driven decisions for your website’s success.
Let’s uncover the truth behind those referral numbers!
Understanding Referral Traffic Sources
Referral traffic can be a goldmine for website growth, but understanding its true value requires careful scrutiny. Not all referral sources are created equal; some might inflate your numbers with low-quality traffic that doesn’t convert. This analysis dives into identifying reliable referral sources and evaluating their contribution to your website’s success.
Top Five Referral Sources and Their Trustworthiness
Let’s imagine a hypothetical e-commerce website selling handcrafted jewelry. Analyzing their referral traffic reveals five key sources: Social Media (Facebook, Instagram, Pinterest), Search Engines (Google, Bing), Email Marketing, Affiliate Programs, and Other Websites (blog mentions, guest posts). The trustworthiness of each source varies significantly. Social media can provide substantial traffic, but its quality can fluctuate depending on campaign effectiveness and audience engagement.
Search engine referrals are generally considered high-quality, indicating users actively searching for products or services like yours. Email marketing offers a targeted approach with a known audience, resulting in potentially high conversion rates. Affiliate programs, while potentially lucrative, require careful vetting of partners to ensure quality traffic. Finally, referral traffic from other websites is highly dependent on the authority and relevance of the referring site.
Referral Traffic Volume Over Three Months
Analyzing traffic volume over time helps determine the consistency and reliability of each source. The following table displays the hypothetical referral traffic for our handcrafted jewelry website over a three-month period:
Source | Month 1 | Month 2 | Month 3 |
---|---|---|---|
Social Media | 500 | 600 | 750 |
Search Engines | 1000 | 1200 | 1500 |
Email Marketing | 200 | 250 | 300 |
Affiliate Programs | 150 | 100 | 200 |
Other Websites | 50 | 75 | 100 |
Note: These figures are hypothetical examples to illustrate data presentation. Real-world data will vary depending on the specific website and its marketing strategies. For instance, a successful social media campaign in Month 3 could explain the increased traffic from that source. Similarly, a less effective affiliate program in Month 2 might be responsible for the decrease in traffic from that source.
Analyzing these fluctuations is crucial for optimizing marketing efforts.
Analyzing Referral Traffic Quality
Raw referral traffic numbers can be misleading. Simply seeing a high volume from a particular source doesn’t guarantee that it’s driving valuable, converting visitors. Analyzing thequality* of your referral traffic is crucial for optimizing your marketing efforts and understanding which sources are truly contributing to your business goals. This involves looking beyond sheer volume and delving into the engagement and conversion rates of visitors arriving from different referral sites.Understanding that referral traffic quality is multifaceted is key.
We need to assess the value each referrer brings, not just their quantity. This means considering the actions visitors take once they arrive on your site. Are they browsing multiple pages? Are they making purchases or signing up for your newsletter? A low conversion rate, even with high referral traffic, signals a quality issue.
Identifying Deceptive Referral Sources
Several referral sources can appear legitimate but ultimately provide low-quality traffic. These sources often inflate your analytics without yielding meaningful results. One common example is referral spam, which artificially inflates referral traffic numbers by sending fake referrals to your website. Another example involves low-quality affiliate programs or partnerships that generate traffic from users who have little interest in your products or services.
This results in high bounce rates and low conversion rates, despite showing up as a significant source of referral traffic. These deceptive sources waste time and resources if you misinterpret their inflated numbers as an indication of genuine interest.
Strategies for Identifying Referral Spam
Identifying and filtering out referral spam is essential for accurate analytics. Several strategies can help:
Effective referral spam detection requires a multi-pronged approach. Ignoring it can lead to skewed data and misinformed marketing decisions. The following strategies, when used together, can significantly improve the accuracy of your referral traffic analysis.
- Examine Referral URLs: Look for unusual or nonsensical URLs in your referral reports. Spam referrals often use long, complex, or obviously fake URLs. These URLs may contain random strings of characters or irrelevant s.
- Analyze Bounce Rate and Session Duration: High bounce rates and extremely short session durations from a specific referral source are strong indicators of spam. Legitimate referrals usually result in more engagement.
- Check for Consistent Low Conversion Rates: If a referral source consistently drives traffic with very low conversion rates, it’s likely low-quality or spam. Genuine referrals should contribute to conversions, sales, or other desired actions.
- Monitor Referral Traffic Over Time: Sudden spikes in traffic from an unfamiliar source warrant closer scrutiny. Legitimate referral traffic usually increases gradually.
- Use Referral Spam Filters: Many analytics platforms offer built-in filters or extensions to block known referral spam sources. Activating these filters can significantly clean up your data.
Investigating Discrepancies in Referral Data
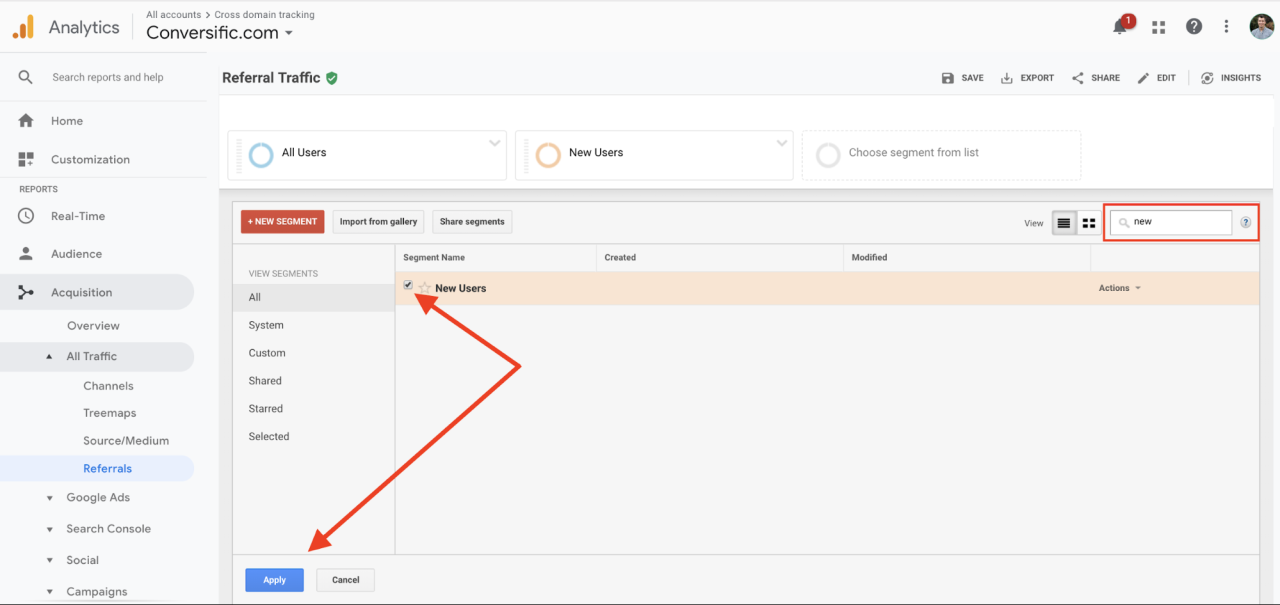
Source: website-files.com
Referral traffic is a crucial metric for understanding your website’s growth and marketing effectiveness. However, the data provided by analytics platforms isn’t always perfectly accurate. Understanding the potential for inaccuracies and developing strategies to investigate discrepancies is vital for making informed business decisions. This section explores common scenarios where referral data can be misleading and provides a structured approach to uncovering the truth behind the numbers.Referral data discrepancies often stem from various sources, leading to inaccurate representations of your website’s referral traffic.
For example, a common problem is “self-referrals,” where internal links within your website are incorrectly categorized as referrals. This inflates the referral numbers and skews the data, making it difficult to identify genuine external sources. Another issue arises from improperly configured tracking codes on partner websites or landing pages, resulting in incomplete or missing referral information. Finally, certain technologies and browser extensions can mask or alter the referral source, providing inaccurate or incomplete data.
These scenarios highlight the need for a systematic approach to investigating inconsistencies.
Identifying Sources of Inaccurate Referral Data
Several scenarios can lead to inaccurate or misleading referral data. Spam referrals, often originating from low-quality or malicious websites, can significantly distort the overall picture. These referrals typically lack meaningful engagement and may even indicate a security vulnerability. Similarly, referrals from social media platforms can sometimes be misattributed, particularly if users access your website through shortened links or embedded content.
Lastly, referrals from search engines themselves can be inconsistently categorized, sometimes appearing as direct traffic or another referral source entirely, depending on how the user accessed your site. These inconsistencies can create significant challenges in accurately interpreting your analytics data.
A Process for Investigating Referral Data Inconsistencies
Investigating inconsistencies begins with comparing your referral data with other key website metrics. For example, if your referral data shows a significant spike in traffic from a specific source, but your conversion rates remain unchanged or even decline, this indicates a potential problem with the referral data’s accuracy. Cross-referencing this discrepancy with other metrics like bounce rate, time on site, and pages per visit can help determine whether the traffic is truly valuable.
Low engagement metrics alongside a high referral count strongly suggest inaccurate or low-quality referrals.
Cross-Referencing Referral Data with Other Analytics Platforms
To verify the accuracy of your referral data, cross-referencing with other analytics platforms is essential. If you use Google Analytics, for instance, comparing your data with data from another platform like Adobe Analytics or Matomo can help identify discrepancies. These discrepancies could be due to differences in tracking methods, data sampling techniques, or even errors in data processing.
This comparison allows for a more holistic understanding of your website’s traffic and identifies potential inconsistencies or biases present in a single analytics platform. A consistent discrepancy across multiple platforms, however, suggests a broader issue needing further investigation, such as problems with your website’s tracking implementation.
Identifying and Addressing Data Manipulation
So, you’ve meticulously analyzed your referral traffic, and something feels…off. The numbers don’t quite add up, and you suspect foul play. Data manipulation in website analytics is a real problem, potentially skewing your understanding of your marketing efforts and leading to poor strategic decisions. Let’s explore why this happens and how to identify and address it.This manipulation can stem from various sources, both internal and external.
Understanding the motivations behind these actions is crucial to effectively combatting them.
Reasons for Referral Data Manipulation
Data manipulation can be driven by a variety of factors, often relating to performance metrics and incentives. For instance, a marketing team might inflate referral traffic to meet targets, while a competitor might attempt to obscure their own marketing activities.
- Meeting performance targets: Inflating referral numbers can make a marketing campaign appear more successful than it actually is, protecting jobs or securing bonuses.
- Concealing poor performance: Manipulating data can mask the ineffectiveness of a specific campaign or strategy.
- Competitive espionage: Competitors might attempt to obscure their marketing activities to prevent others from replicating their success.
- Fraudulent activity: In more extreme cases, data manipulation might be part of a larger scheme to defraud advertisers or investors.
Identifying Data Manipulation: A Step-by-Step Approach
Suspecting data manipulation requires a systematic approach. The following flowchart Artikels a process for investigating and addressing these issues. Remember, thorough investigation is key. Imagine a flowchart with the following steps:
1. Start
Notice inconsistencies in referral data.
2. Compare Data Sources
Cross-reference referral data with other analytics platforms and internal data.
3. Analyze Referral Patterns
Look for unusual spikes, drops, or patterns in referral traffic.
4. Investigate Suspicious Referrers
Deep dive into the websites listed as referral sources.
5. Examine Website Code
Check for any unusual or suspicious code snippets that might be manipulating referral data.
6. Data Manipulation Confirmed?
If yes, proceed to remediation; if no, review data collection and analysis methods.
Ever wonder if your analytics are truly reflecting reality? Sometimes referral traffic can be misleading, especially when you’re trying to track the effectiveness of specific campaigns. For example, if you’re heavily invested in video marketing, you might find it helpful to read this great article on getting it on with youtube to understand how YouTube’s reporting interacts with your overall analytics.
Understanding these nuances is crucial to accurately assess if your referral traffic is painting a true picture of your website’s performance and where your visitors are really coming from.
7. Remediation
Address the root cause of the manipulation, whether it’s a technical issue, malicious activity, or internal process problem.
8. Implement Preventative Measures
Strengthen data security and implement measures to prevent future manipulation.
9. End
Referral data is accurate and reliable.
Techniques Used to Mask Referral Sources
Several techniques can be employed to mask the true origin of website traffic. These range from relatively simple methods to more sophisticated ones that require technical expertise.
- UTM Parameter Manipulation: Modifying or removing UTM parameters designed to track the source of traffic.
- Referrer Spam: Fake referrals from non-existent or malicious websites flooding analytics data.
- JavaScript Manipulation: Using JavaScript code to override or alter the referrer information sent to analytics platforms.
- Proxy Servers: Routing traffic through proxy servers to mask the original IP address and referrer information.
- Cloaking: Presenting different content to search engines and users, thus obscuring the true source of traffic.
Improving Referral Traffic Measurement
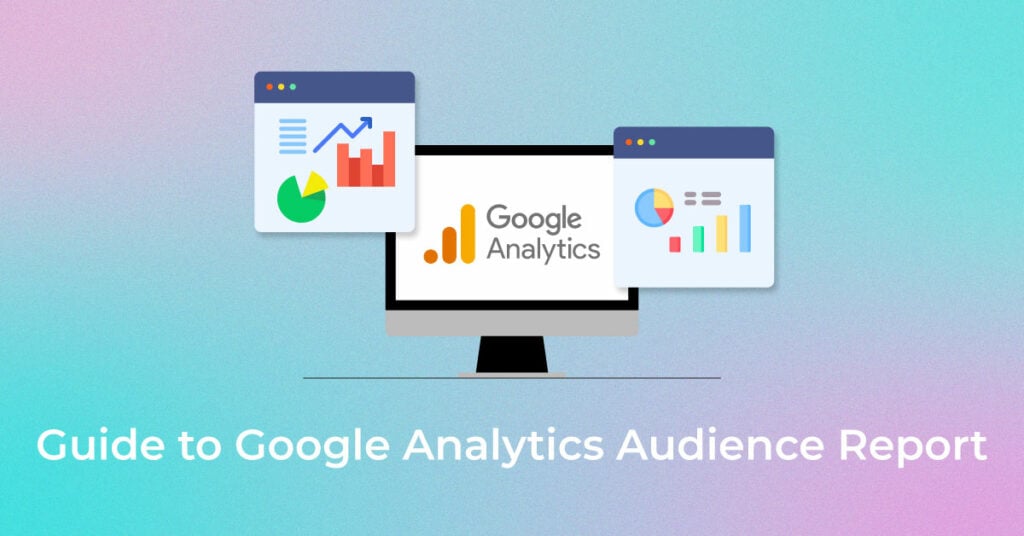
Source: infidigit.com
So, you’ve identified that your referral traffic data might be… less than perfect. That’s a common problem, and thankfully, there are steps you can take to significantly improve the accuracy of your measurements. By implementing better tracking and utilizing the right tools, you can gain a much clearer picture of where your valuable referral traffic is actually coming from.
This will allow for more effective marketing strategies and a better understanding of your audience.Accurate referral traffic measurement is crucial for optimizing your marketing efforts. Inaccurate data leads to misguided strategies and wasted resources. By understanding how to properly track and interpret referral data, you can make informed decisions about where to focus your energy and budget. This section will Artikel best practices for improving the reliability of your referral data, leading to more effective marketing decisions.
Best Practices for Accurate Referral Traffic Tracking
Implementing robust tracking mechanisms is the cornerstone of accurate referral data. This involves utilizing UTM parameters effectively, regularly auditing your referral sources, and carefully examining any discrepancies. Ignoring these practices can lead to a skewed understanding of your referral traffic, hindering your ability to optimize campaigns and allocate resources effectively. For instance, a company might invest heavily in a particular referral source based on flawed data, only to discover later that the actual contribution was significantly lower.
Implementing Proper Tagging and Tracking Mechanisms
Proper tagging involves using UTM (Urchin Tracking Module) parameters appended to your URLs. These parameters allow you to track specific campaigns, sources, and mediums. For example, a link to your website from a social media post might include UTM parameters like `utm_source=facebook`, `utm_medium=social`, and `utm_campaign=summer_sale`. This granular level of tracking provides a detailed breakdown of your referral traffic, allowing you to pinpoint the most effective channels.
Without proper UTM tagging, all traffic from Facebook would be lumped together, obscuring valuable insights into individual campaign performance. Failure to correctly implement these tags results in inaccurate attribution and a blurred understanding of marketing ROI.
Tools and Techniques for Improving Referral Data Reliability
Several tools and techniques can help enhance the reliability of your referral data. These range from using analytics platforms with robust tracking capabilities to employing dedicated referral tracking software. Utilizing multiple tools and cross-referencing data helps identify and mitigate potential inaccuracies. For example, comparing data from Google Analytics with data from your CRM can highlight inconsistencies, potentially indicating data manipulation or other issues.
Relying solely on a single source of data increases the risk of inaccurate conclusions and inefficient resource allocation.
- Google Analytics: Offers robust tracking capabilities, including advanced segmentation and custom reports to analyze referral traffic in detail.
- Referral tracking software: Specialized tools provide deeper insights into referral traffic, often with features beyond standard analytics platforms.
- Data visualization tools: Tools like Tableau or Power BI can help visualize referral data, making it easier to identify patterns and anomalies.
- Regular data audits: Conducting regular audits of your referral data helps identify and correct inaccuracies before they significantly impact your marketing decisions.
Visualizing Referral Traffic Data
Understanding your website’s referral traffic is crucial for optimizing marketing strategies. Raw data, however, often lacks the clarity needed to spot trends or identify problematic areas. Visualizing this data transforms complex numbers into easily digestible insights, allowing for quicker identification of opportunities and challenges. This process is vital for both technical and non-technical stakeholders.Visualizing referral traffic allows for a quick and comprehensive understanding of the sources driving traffic to your website.
It’s a critical step in moving beyond simply knowing
- which* sources are sending traffic and towards understanding
- how much* and
- what kind* of traffic they are sending. This understanding is essential for informed decision-making regarding marketing budget allocation and campaign optimization.
A Visual Representation of Referral Traffic Distribution
A highly effective visualization for referral traffic would be a donut chart. The entire donut represents your total referral traffic. Each segment within the donut would represent a different referral source (e.g., Facebook, Google, specific partner websites, etc.). The size of each segment would be proportional to the percentage of total referral traffic it contributes. For instance, if Facebook contributes 40% of your referral traffic, its segment would occupy 40% of the donut’s area.
Adding labels to each segment clearly identifying the source and its percentage contribution further enhances understanding. A legend could also be included for clarity, especially if there are many sources. The color coding of the segments could be consistent with your brand or chosen marketing materials for easy recognition. This allows for immediate comparison of the relative contribution of each referral source at a glance.
The Importance of Visualization for Pattern and Anomaly Identification, Is your analytics referral traffic lying to you
Visualizations significantly enhance the ability to identify patterns and anomalies within referral traffic data. For example, a sudden drop in traffic from a specific source immediately stands out in a chart, prompting investigation into the cause. Similarly, a consistently underperforming source becomes apparent, allowing for a reassessment of the marketing strategy related to that source. Trends, such as seasonal fluctuations or the impact of specific marketing campaigns, are also much easier to spot visually than in a spreadsheet.
Anomalies, like a sudden spike in traffic from an unexpected source, could indicate either a successful unexpected marketing effort or a potential issue, such as a malicious link.
Communicating Findings to Non-Technical Audiences
Visualizations are exceptionally useful for communicating complex data to non-technical audiences. A well-designed donut chart, for instance, clearly shows the relative contribution of each referral source without requiring any deep understanding of analytics. Accompanying the chart with concise, clear explanations, focusing on the key takeaways (e.g., “Facebook is our most successful referral source, driving 40% of our traffic,” or “Traffic from Source X has decreased significantly this month, requiring investigation.”), further simplifies the communication.
Avoiding technical jargon and using plain language ensures that the message is easily understood and actionable, regardless of the audience’s technical expertise.
Summary: Is Your Analytics Referral Traffic Lying To You
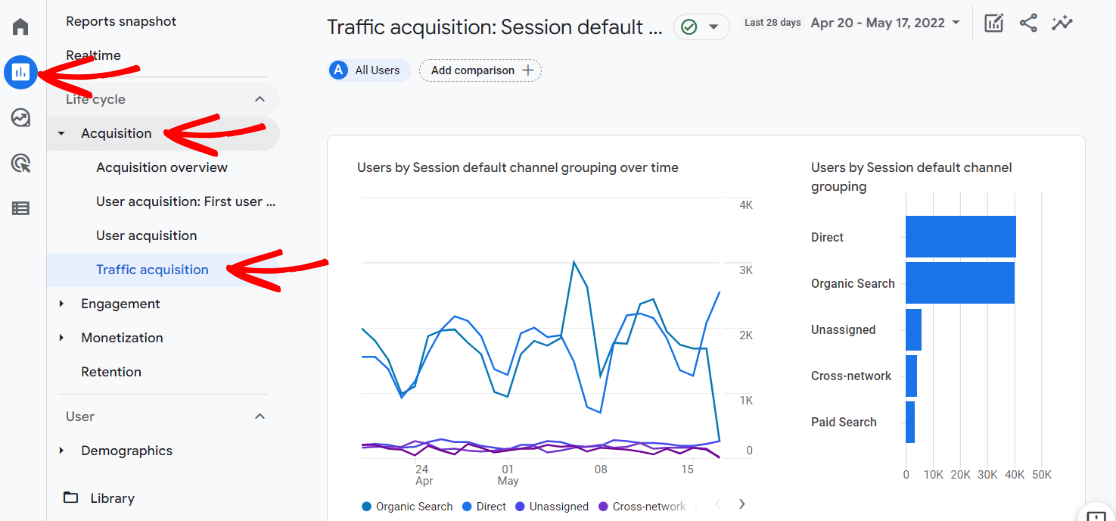
Source: monsterinsights.com
So, is your referral traffic truly representative of your audience? By understanding the potential pitfalls of referral data and employing the strategies discussed here, you can significantly improve the accuracy of your analytics. Don’t let misleading numbers derail your website’s growth. Become a more informed website owner, equipped to analyze your data critically and make better decisions based on reliable insights.
Remember, accurate data is the foundation of effective marketing and website optimization. Start investigating your referral traffic today – you might be surprised by what you find!
Detailed FAQs
What are some common examples of referral spam?
Common examples include referrals from suspicious domains, often containing gibberish or irrelevant s. These sources often show unusually high traffic volumes with little to no actual engagement on your site.
How often should I review my referral traffic data?
Regularly reviewing your referral data, ideally weekly or monthly, allows you to catch anomalies early and investigate potential issues before they significantly impact your analytics.
Can I trust referral data from social media platforms?
While generally reliable, social media referral data can sometimes be incomplete or inaccurate due to various factors, including how links are shared and tracked. Cross-referencing with other metrics is advisable.
What tools can help me visualize my referral traffic data?
Many analytics platforms offer built-in visualization tools (like charts and graphs). Additionally, tools like Google Data Studio and Tableau can help create more customized and sophisticated visualizations.